The Mapping Cancer Markers team discusses the past (lung cancer), present (ovarian cancer), and future (sarcoma) of the project in this comprehensive update.
Background
The Mapping Cancer Markers (MCM) project was designed to identify the markers associated with various types of cancer, and by refining the process of identifying these markers, identify such biomarkers for other diseases more efficiently. We aimed to analyze multiple cancer datasets in order to identify potential biomarkers for these cancers, which could eventually help scientists and physicians detect cancers earlier and create personalized treatments. The first three datasets in MCM plan are lung, ovarian, and sarcoma, representing the past, present and future of MCM. Lung processing is complete. Ovarian marker processing is underway, but nearing completion. We are now preparing for a switch to sarcoma.
Processing a dataset on World Community Grid over months and years produces a huge amount of data, and this data is not directly usable, but must then be collated, filtered, and analyzed in different ways. We have been focusing on this post-processing step in our lab.
In this update, we will mainly discuss some of the work done with the processed lung dataset, but first, we will take a quick glance at the future.
Final preparations for sarcoma dataset
The upcoming sarcoma dataset will be MCM’s most complex dataset to-date. It contains potential biomarkers drawn from multiple sources: measurements of RNA, DNA, and protein activity, mutations, and other biological modalities.
With such detailed information about each sample in the dataset, it took some effort to reduce the dataset and result sizes to practical levels. We are currently testing work units of our draft dataset and are planning the work.
A future update will announce the launch of a new phase of the MCM project, focusing on sarcoma and provide more details.
Results from the lung dataset
The biomarkers in the MCM lung dataset measure the activity of thousands of genes. Collectively, these biomarkers cover most of the human genome. The majority of MCM lung work processed on World Community Grid surveyed signatures randomly drawn from the entire set of biomarkers. A shorter, second phase of MCM lung drew signatures from optimized subsets of those biomarkers.
The contribution of compute cycles to the project was extraordinary. World Community Grid members processed 4.5 trillion candidate lung cancer signatures in the main phase of MCM lung, 220 billion in an initial experimental phase, and 1.6 trillion signatures in the optimizing phase.
We will discuss some findings from the main phase of MCM lung in this update.
The question of signature size
MCM lung surveyed signatures of multiple sizes. Sizes varied from 5 biomarkers to 100, with the greatest focus on signatures in the range of 10-20 biomarkers. For a cancer signature to succeed in clinical use, signature size is a compromise between diagnostic power, complexity, and cost. Every biomarker can potentially add diagnostic information to a signature, increasing accuracy, but too many biomarkers can also add noise and unnecessarily increase cost and complexity for practical use in the clinic.
The figure below shows the effect of signature size on peak accuracy. For almost any size, a signature built from randomly-chosen biomarkers will have poor accuracy, but by testing enough such signatures, and then looking at the accuracy of the top fraction (say, the top 0.01%), we see the effect made by signature size. Carefully engineered signatures should achieve the same accuracy using fewer biomarkers.
Figure 1A
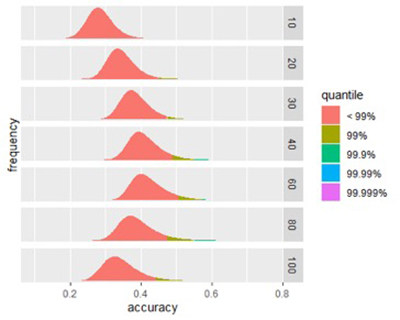
Figure 1B
Figures 1A and 1B: Size affects a signature’s potential accuracy. (A) The score distribution from successful signatures of different sizes. (B) A closer look at the effect of size of score accuracy. Peak accuracy of is found in signatures between 40-80 biomarkers.
Which biomarkers are most successful?
In the main phase of MCM lung, signatures were built from biomarkers chosen randomly from the dataset. As such, every biomarker had an equal chance of appearing in each new signature. This does not mean, though, that all biomarkers are equally useful – as we said above, a random signature will most likely have low accuracy. If, however, we take only the most accurate fraction of signatures, and see which biomarkers they contain, we see that a few biomarkers appear frequently, and that the rest are relatively rare. (We may even notice patterns in ways that certain groups of biomarkers appear together, as we discussed in a previous update.) We can determine then how effective or useful each biomarker is from how often it appears in these top signatures.
Figure 2
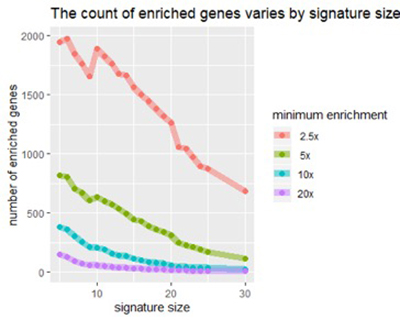
After analyzing the full set of MCM lung results, we can confirm an effect that we had noticed in earlier, preliminary studies: the effectiveness of each biomarker depends on the signature size, affecting each biomarker differently. The figure below illustrates the effect for some of the top-ranked biomarkers.
Figure 3
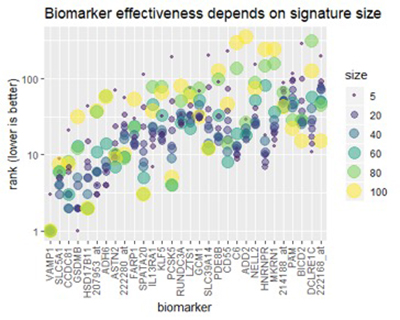
Pathway enrichment among the top biomarkers
To get a higher-level view of the biomarkers discovered in the lung dataset, we examined them from the pathway perspective. A pathway is a group of genes that cooperate to perform the same biological function. We fed lists of top-1% biomarkers into our lab’s pathDIP database [1], [2]. pathDIP is a comprehensive, integrated database of known pathways (signaling cascades), and given a list of genes, it will find all pathways associated with any gene in the list. Most usefully, it will measure the enrichment of each pathway in your gene list – the degree to which pathway has an above-average connection to your list. Using such analysis, we aim to find biologically meaningful interpretation of our identified biomarkers.
The figure below shows the results from pathDIP.
Figure 4
Across a large number of signature sizes, pathDIP consistently found five pathways enriched in our gene lists:
- Cyclophosphamide Pathway, Pharmacodynamics
- Ifosfamide Pathway, Pharmacodynamics
- Ethanol oxidation
- Fatty Acid Omega oxidation
- Oxidative Stress Regulatory Pathway (Erythrocyte)
All five enriched pathways relate to metabolism, meaning the breakdown of chemicals in the body. Curiously, the first two pathways relate specifically to metabolism of chemotherapy drugs, Cyclophosphamide and Ifosfamide. The last three relate to either oxidation or the prevention of oxidative stress (free radicals) in red blood cells.
Using the Gene Ontology Resource to describe top biomarkers
We can get a related view from the Gene Ontology Resource (GO). GO categorizes each gene from three different perspectives: biological process, molecular function, and cellular component. The figures below show terms in GO categories that appear frequently in top 1% biomarkers.
Figure 5
Figure 6
Figure 7
Many of the terms reflect the themes found in pathways: oxidation, alcohol, and red-blood-cell chemistry.
Looking ahead
We are in the process of expanding and combining multiple additional analyses of the main-phase lung data, and substantial analyses of the second phase lung results. After that, the ovarian data awaits. For ovarian, some of the same techniques will apply, but some will need to be adapted, and some we’ll need to develop.
In short, the MCM project will keep us busy for a long time. In the meantime, we would like to thank you for your interest and for your generous donation of computing power to this and other World Community Grid projects. We will provide updates more frequently now.
Additional results
Over the last two years, we have published several original manuscripts and multiple applications using our tools and programs, many of which we have been using to increase the value of MCM analyses:
- PMID: 31583635Kennedy, S., Jarboui, M-A, Srihari, S, Raso, C, Bryan, K, Dernayka, L, Charitou. T, Bernal-Llinares, M, Herrera-Montavez, C, Krstic, A, Matallanas, D, Kotlyar, M, Jurisica, I, Curak, J, Wong, V, Stagljar, I, LeBihan, T, Imrie, L, Pillai, P, Lynn, M, Fasterius, E, Szigyarto, C. A-K, Breen, J, Kiel, C, Serrano, L, Rauch, N, Rukhlenko, O, Kholodenko, B, Iglesias-Martinez, L, Ryan, C, Pilkington, R, Cammareri, P, Sansom, O, Shave, S, Auer, M, Horn, N, Klose, F, Ueffing, M, Boldt, K, Lynn, D, Kolch, W, Extensive Rewiring of the EGFR Network in Colorectal Cancer Cells Expressing Transforming Levels of KRASG13D, Nat Commun, 2019. In press.
- Enfield, K.S.S., Marshall, E.A., Anderson, C., Ng, K.W., Rahmati, S, Xu, Z. Fuller, M., Milne, K., Lu, D., Shi, R., Rowbotham, D. A., Becker-Santos, D.D., Johnson, F.D., English, J.C., MacAulay, C.E., Lam, S., Lockwood, W.W., Chari, R., Karsan, A., Jurisica, I., Lam, W.L., Epithelial tumor suppressor ELF3 is a lineage-specific amplified oncogene in lung adenocarcinoma, Nat Commun,10(1):5438, 2019. doi:10.1038/s41467-019-13295-y
- Rahmati, S., Abovsky, M., Pastrello, C., Kotlyar, M., Lu, R., Cumbaa, C.A., Rahman, P., Chandran, V. and Jurisica, I. pathDIP 4: An extended pathway annotations and enrichment analysis resource for human, model organisms and domesticated species, Nucl Acids Res, In press. 2019. https://doi.org/10.1093/nar/gkz989
- Holzinger A, Haibe-Kains B, Jurisica I. Why imaging data alone is not enough: AI-based integration of imaging, omics, and clinical data, Eur J Nucl Med Mol Imaging. 2019 Jun 15. doi: 10.1007/s00259-019-04382-9.
- Monette A, Bergeron D, Ben Amor A, Meunier L, Caron C, Mes-Masson AM, Kchir N, Hamzaoui K, Jurisica I, Lapointe R. Immune-enrichment of non-small cell lung cancer baseline biopsies for multiplex profiling define prognostic immune checkpoint combinations for patient stratification, J Immunother Cancer, 7(1):86, 2019. doi: 10.1186/s40425-019-0544-x
- Monette A, Morou A, Al-Banna NA, Rousseau L, Lattouf JB, Rahmati S, Tokar T, Routy JP, Cailhier JF, Kaufmann DE, Jurisica I, Lapointe R. Failed immune responses across multiple pathologies share pan-tumor and circulating lymphocytic targets, J Clin Invest, 129(6):2463-2479, 2019. doi: 10.1172/JCI125301
- Mohammed Ali Z, Tokar T, Batruch I, Reid S, Tavares-Brum A, Yip P, Cardinal H, Hébert MJ, Li Y, Kim SJ, Jurisica I, John R, Konvalinka A. Urine Angiotensin II Signature Proteins as Markers of Fibrosis in Kidney Transplant Recipients, Transplantation,103(6):e146-e158, 2019. doi: 10.1097/TP.0000000000002676.
- Kaufmann KB, Garcia-Prat L, Liu Q, Ng SWK, Takayanagi SI, Mitchell A, Wienholds E, van Galen P, Cumbaa CA, Tsay MJ, Pastrello C, Wagenblast E, Krivdova G, Minden MD, Lechman ER, Zandi S, Jurisica I, Wang JCY, Xie SZ, Dick JE. A stemness screen reveals C3orf54/INKA1 as a promoter of human leukemia stem cell latency, Blood, 133(20):2198-2211, 2019. doi: 10.1182/blood-2018-10-881441
- Mandilaras, V, Garg, S, Cabanero, M, Tan, Q, Pastrello, C, Burnier, J, Karakasis, K, Wang, L, Dhani, NC, Butler, MO, Bedard, PL, Siu, LL, Clarke, B, Shaw, PA, Stockley, T, Jurisica, I, Oza, AM. TP53 mutations in high grade serous ovarian cancer and impact on clinical outcomes: a comparison of next generation sequencing and bioinformatics analyses. Int J Gyn Cancer, Jan 18. pii: ijgc-2018-000087. doi: 10.1136/ijgc-2018-000087.
- del Toro N, Duesbury M, Koch M, Perfetto L, Shrivastava A, Ochoa D, Wagih O, Piñero J, Kotlyar M, Pastrello C, Beltrao P, Furlong LI, Jurisica I, Hermjakob H, Orchard S, Porras P. Capturing variation impact on molecular interactions in the IMEx Consortium mutations data set. Nat Commun, 10(1): 10, 2019.
- Li L, Guturi KKN, Gautreau B, Patel PS, Saad A, Morii M, Mateo F, Palomero L, Barbour H, Gomez A, Ng D, Kotlyar M, Pastrello C, Jackson HW, Khokha R, Jurisica I, Affar EB, Raught B, Sanchez O, Alaoui-Jamali M, Pujana MA, Hakem A, Hakem R., Ubiquitin ligase RNF8 suppresses Notch signaling to regulate mammary development and tumorigenesis, J Clin Invest, 128(10):4525-4542, 2018. doi: 10.1172/JCI120401
- Kotlyar, M., Pastrello, C., Malik, Z., Jurisica, I., IID 2018 update: context-specific physical protein-protein interactions in human, model organisms and domesticated species. Nucleic acids research, 47(D1):D581-D589, 2019.
- Endisha, H., Rockel, J., Jurisica, I., Kapoor, M., The complex landscape of microRNAs in articular cartilage: biology, pathology, and therapeutic targets, JCI Insight. 3(17):e121630, 2018.
- Singh, M., Venugopal, C., Tokar, T., McFarlane, N., Subapanditha, M. K., Qazi, M., Bakhshinyan, D., Vora, P., Murty, N., Jurisica, I., Singh, S. K., Therapeutic targeting of the pre-metastatic stage in human brain metastasis, Cancer Res, 2018. ePub 2018/07/11. DOI: 10.1158/0008-5472.CAN-18-1022.
- Wen, B., Tokar, T., Taibi, A., Chen, J., Jurisica, I., Comelli, E. M. Citrobacter rodentium alters the mouse colonic miRNome, Genes and Immunity, 2018. In press. ePub 2018/05/08. Doi: 10.1038/s41435-018-0026-z
- Jean-Quartier C, Jeanquartier F, Jurisica I, Holzinger A, In silico cancer research towards 3R. BMC Cancer, 18(1):408, 2018
- Sivade Dumousseau M, Alonso-López D, Ammari M, Bradley G, Campbell NH, Ceol A, Cesareni G, Combe C, De Las Rivas J, Del-Toro N, Heimbach J, Hermjakob H, Jurisica I, Koch M, Licata L, Lovering RC, Lynn DJ, Meldal BHM, Micklem G, Panni S, Porras P, Ricard-Blum S, Roechert B, Salwinski L, Shrivastava A, Sullivan J, Thierry-Mieg N, Yehudi Y, Van Roey K, Orchard S. Encompassing new use cases - level 3.0 of the HUPO-PSI format for molecular interactions. BMC Bioinformatics, 19(1):134, 2018.
- Minatel BC, Martinez VD, Ng KW, Sage AP, Tokar T, Marshall EA, Anderson C, Enfield KSS, Stewart GL, Reis PP, Jurisica I, Lam WL., Large-scale discovery of previously undetected microRNAs specific to human liver. Hum Genomics, 12(1):16, 2018.
- Tokar T, Pastrello C, Ramnarine VR, Zhu CQ, Craddock KJ, Pikor L, Vucic EA, Vary S, Shepherd FA, Tsao MS, Lam WL, Jurisica I Differentially expressed microRNAs in lung adenocarcinoma invert effects of copy number aberrations of prognostic genes. Oncotarget. 9(10):9137-9155, 2018.
- Paulitti A, Corallo D, Andreuzzi E, Bizzotto D, Marastoni S, Pellicani R, Tarticchio G, Pastrello C, Jurisica I, Ligresti G, Bucciotti F, Doliana R, Colladel R, Braghetta P, Di Silvestre A, Bressan G, Colombatti A, Bonaldo P, Mongiat M. The ablation of the matricellular protein EMILIN2 causes defective vascularization due to impaired EGFR-dependent IL-8 production affecting tumor growth, Oncogene, 37(25): 3399-3414, 2018.
- Tokar, T., Pastrello, C., Rossos, A., Abovsky, M., Hauschild, A.C., Tsay, M., Lu, R., Jurisica, I. mirDIP 4.1 – Integrative database of human microRNA target predictions, Nucl Acids Res, D1(46): D360-D370, 2018.
- Pastrello C, Kotlyar M, Jurisica I., Informed Use of Protein-Protein Interaction Data: A Focus on the Integrated Interactions Database (IID). Methods Mol Biol., 2074:125-134, 2020. doi: 10.1007/978-1-4939-9873-9_10.
- Hauschild, A-C, Pastrello, C, Kotlyar, M and Jurisica, I. Protein-protein interaction data, their quality, and major public databases. Ed. N. Przulj. Analyzing Network Data in Biology and Medicine, An Interdisciplinary Textbook for Biological, Medical and Computational Scientists, Cambridge University Press, Cambridge, UK, pp.151-192, 2019. ISBN 978-1-108-43223-8. DOI: 10.1017/978110837770
- Wong, S., Pastrello, C., Kotlyar, M., Faloutsos, C., Jurisica, I. SDREGION: Fast spotting of changing communities in biological networks. Proceedings of the ACM SIGKDD International Conference on Knowledge Discovery and Data Mining, pp. 867-875, 2018.
- Kotlyar, M., Pastrello, C., Rossos, A., Jurisica, I. Protein-protein interaction databases. In: Ranganathan, S., Nakai, K., Schönbach C. and Gribskov, M. (eds.), Encyclopedia of Bioinformatics and Computational Biology, vol. 1, pp. 988–996. Oxford: Elsevier, 2018.
- Rahmati, S., Pastrello, C., Rossos, A., Jurisica, I. Two Decades of Biological Pathway Databases: Results and Challenges, In: Ranganathan, S., Nakai, K., Schönbach C. and Gribskov, M. (eds.), Encyclopedia of Bioinformatics and Computational Biology, vol. 1, pp. 1071–1084. Oxford: Elsevier, 2018.
- Hauschild, AC, Pastrello, C., Rossos, A., Jurisica, I. Visualization of Biomedical Networks, In: Ranganathan, S., Nakai, K., Schönbach C. and Gribskov, M. (eds.), Encyclopedia of Bioinformatics and Computational Biology, vol. 1, pp. 1016–1035. Oxford: Elsevier, 2018.
Other news
In other news, we have been able to secure several grants to enable funding for the project, including:
- Novel methods for integrative computational biology from Natural Sciences and Engineering Council of Canada,
- Interactome mapping of disease-related proteins using split intein-mediated protein ligation (SIMPL) from Genome Canada,
- The Next Generation Signalling Biology Platform from Ontario Research Funds
- Canadian Institutes of Health in collaboration with European funding agencies
Importantly, we also had a chance to host two World Community Grid and MCM supporters at our institute[3]. Dylan Bucci, a Sisler High School student, and network and cybersecurity teacher Robert Esposito visited our research institution to meet with us and our collaborators, the scientists who use the results from the analysis. It was interesting for us to learn about their motivation, and for them to experience direct and indirect research links to MCM.
Thank you for all the contributed computing power that makes this research possible.
The MCM Team
[1] Rahmati, S., Abovsky, M., Pastrello, C., Jurisica, I. pathDIP: An annotated resource for known and predicted human gene-pathway associations and pathway enrichment analysis. Nucl Acids Res 45(D1): D419-D426, 2017.
[2] Rahmati, S., Abovsky, M., Pastrello, C., Kotlyar, M., Lu, R., Cumbaa, C.A., Rahman, P., Chandran, V. and Jurisica, I. pathDIP 4: An extended pathway annotations and enrichment analysis resource for human, model organisms and domesticated species, Nucl Acids Res, In press. 2019. https://doi.org/10.1093/nar/gkz989
[3] CTV News Interview on IBM World Community Grid, Mapping Cancer Markers, May 3, 2019