Results from the first stage of the Mapping Cancer Markers project are helping the researchers identify the markers for lung cancer, as well as improve their research methodology as they move on to analyze other cancers.
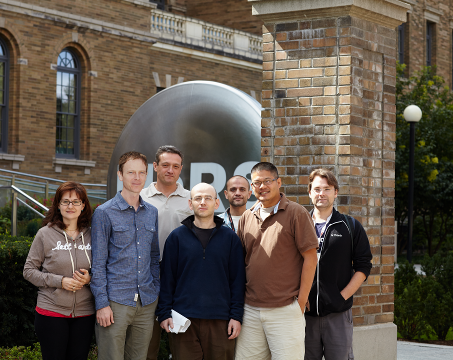
Once again, the Mapping Cancer Markers (MCM) team would like to extend a huge thank you to the World Community Grid members. Although we publish this thank you each update, we are truly grateful for your contribution to this project.
The MCM project has continued to process lung cancer data, exploring fixed-length random gene signatures. This long stage of the project is nearly over, and we are preparing to transition our focus to a narrower set of genes of interest. Target genes will be chosen by a process combining statistics from the initial results, with pathway and biological-network analysis.
Analytics
In our previous update, we reported the adoption of a new package, the IBM® InfoSphere® Streams real-time analytics platform, to process our World Community Grid data. The majority of our work since the last update has concentrated on continued development and expansion of our Streams system in order to handle the incoming data more robustly and efficiently.
There are two main reasons why stream-processing design is better for processing MCM results than a batch-computing approach. One reason relates to the nature of World Community Grid: a huge computing resource that continuously consumes work units and produces compute results. Data is best processed as it arrives, to avoid backlogs or storage limitations.
Importantly, as we transition to the new focus, this enables us to make the process of designing new work units based on partial results more effective. MCM will soon focus on genes of interest revealed by our broad survey of gene-signature space in the first stage. To narrow the focus, we will take an iterative approach, where we design small batches of work units (e.g., 100,000 units), submit them to World Community Grid, analyze the results, and then incorporate the new analysis into designing the next batch. In this way, we will slowly converge towards the answers we are seeking. Because of the continuous nature of the MCM project, and the volume of data we receive on a daily basis, it is imperative that our analysis system processes results quickly enough to generate the next set of work units.
New stage in lung cancer signature discovery
The MCM project has continued to process lung cancer data, exploring random fixed-length signatures of between 5 and 25 biomarkers. This computational component of the “landscape” stage is winding down, and we are preparing to transition our focus to a narrower set of genes of interest. Target genes will be selected by integrating results from several methods, carefully combining statistics from the initial results with pathway and biological-network analysis.
Network analysis/integration of pathway knowledge
One of the most exciting (and crucial) parts of this project is the integration of other research to help understand the results we are collecting. We already know that in most cancers no single biomarker is sufficient, we can find thousands of clinically-relevant signatures, and, most importantly, many seemingly weak markers when combined with others provide highly useful information. Therefore, we have been trying to find these “best supporting actors” and then the best signatures through “integrative network analysis”.
We know that disease is more accurately described in terms of altered signaling cascades (pathways): higher-level patterns composed of multiple genes in a biological network. A pathway can be defined as a series of reactions (“steps”) that result in a certain biochemical process. For example, one could consider the electrical and mechanical systems in a car as a set of interrelated pathways. These systems are important for the overall function of the car; however, some are clearly more important than others. In the same way, a particular cancer occurrence could have a single catastrophic cause (a missing engine block) or smaller, multiple causes affecting the same system (e.g., the bolts holding the exhaust system together).
Around the world, researchers are continually finding, publishing and curating biological pathways and their building blocks (protein interactions). We are taking this information and applying it to high-scoring genes and gene signatures identified from Mapping Cancer Marker results. For example, if the first part of our landscape study identified a certain gene as a potential target, we can see via our network analysis (NAViGaTOR) as well as other external sources if that same gene is involved in known pathways. We can then gather information about those pathways and refine our findings by resubmitting work units to World Community Grid. In essence, we are identifying genes of interest by combining top-scoring genes with pathway and network context. Those investigations will continue to refine our search space and converge on better and better solutions. Below, we list some examples of this work, but especially Kotlyar et al., Nature Methods, 2015 work provides comprehensive in silico prediction of these signaling cascades. Wong et al., Proteomics, 2015 introduces systematic approach to derive important information about cancer-related structures in these networks. Fortney et al., PLoS Computational Biology uses results of this work to identify potential new treatment options for lung cancer.
Transition to the targeted stage
We expect a gradual and seamless transition to the new stage of MCM, with no interruption in the supply of work units, and no changes to the visualization or code. Both stages will overlap for a period as the last statistics from the first stage are gathered, and the initial, targeted work units are sent out. Average work unit run-time should remain the same. The consistency of run-times should remain the same or improve.
Some related published work
Hoeng J, Peitsch MC, Meyer, P. and Jurisica, I. Where are we at regarding Species Translation? A review of the sbv IMPROVER Challenge, Bioinformatics, 2015. In press.
Fortney, K., Griesman, G., Kotlyar, M., Pastrello, C., Angeli, M., Tsao, M.S., Jurisica, I. Prioritizing therapeutics for lung cancer: An integrative meta-analysis of cancer gene signatures and chemogenomic data, PLoS Comp Biol, 2015, In Press.
Kotlyar M., Pastrello C., Pivetta, F., Lo Sardo A., Cumbaa, C., Li, H., Naranian, T., Niu Y., Ding Z., Vafaee F., Broackes-Carter F., Petschnigg, J., Mills, G.B., Jurisicova, A., Stagljar, I., Maestro, R., & Jurisica, I. In silico prediction of physical protein interactions and characterization of interactome orphans, Nat Methods, 12(1):79-84, 2015.
Vucic, E. A., Thu, K. T., Pikor, L. A., Enfield, K. S. S., Yee, J., English, J. C., MacAulay, C. E., Lam, S., Jurisica, I., Lam, W. L. Smoking status impacts microRNA mediated prognosis and lung adenocarcinoma biology, BMC Cancer, 14: 778, 2014. E-pub 2014/10/25
Lalonde, E., Ishkanian, A. S., Sykes, J., Fraser, M., Ross-Adam, H., Erho, N., Dunning, M., Lamb, A.D., Moon, N.C., Zafarana, G., Warren, A.Y., Meng, A., Thoms, J., Grzadkowski, M.R., Berlin, A., Halim, S., Have, C.L., Ramnarine, V.R., Yao, C.Q., Malloff, C.A., Lam, L. L., Xie, H., Harding, N.J., Mak, D.Y.F., Chu1, K. C., Chong, L.C., Sendorek, D.H., P’ng, C., Collins, C.C., Squire, J.A., Jurisica, I., Cooper, C., Eeles, R., Pintilie, M., Pra, A.D., Davicioni, E., Lam, W. L., Milosevic, M., Neal, D.E., van der Kwast, T., Boutros, P.C., Bristow, R.G., Tumour genomic and microenvironmental heterogeneity for integrated prediction of 5-year biochemical recurrence of prostate cancer: a retrospective cohort study. Lancet Oncology. 15(13):1521-32, 2014.
Dingar, D., Kalkat, M., Chan, M. P-K, Bailey, S.D., Srikumar, T., Tu, W.B., Ponzielli, R., Kotlyar, M., Jurisica, I., Huang, A., Lupien, M., Penn, L.Z., Raught, B. BioID identifies novel c-MYC interacting partners in cultured cells and xenograft tumors, Proteomics, pii: S1874-3919(14)00462-X, 2014. doi: 10.1016/j.jprot.2014.09.029
Wong, S. W. H., Cercone, N., Jurisica, I. Comparative network analysis via differential graphlet communities, Special Issue of Proteomics dedicated to Signal Transduction, Proteomics, 15(2-3):608-17, 2015. E-pub 2014/10/07. doi: 10.1002/pmic.201400233
Berlin, A., Lalonde, E., Sykes, J., Zafarana, G., Chu, K.C., Ramnarine, V.R., Ishkanian, A., Sendorek, D.H.S., Pasic, I., Lam, W.L., Jurisica, I., van der Kwast, T., Milosevic, M., Boutros, P.C., Bristow, R.G.. NBN Gain Is Predictive for Adverse Outcome Following Image-Guided Radiotherapy for Localized Prostate Cancer, Oncotarget, 3:e133, 2014.
Lapin, V., Shirdel, E., Wei, X., Mason, J., Jurisica, I., Mak, T.W., Kinome-wide screening of HER2+ breast cancer cells for molecules that mediate cell proliferation or sensitize cells to trastuzumab therapy, Oncogenesis, 3, e133; doi:10.1038/oncsis.2014.45, 2014.
Tu WB, Helander S, Pilstål R, Hickman KA, Lourenco C, Jurisica I, Raught B, Wallner B, Sunnerhagen M, Penn LZ. Myc and its interactors take shape. Biochim Biophys Acta. pii: S1874-9399(14)00154-0.