The Mapping Cancer Markers researchers are analyzing the results of the lung cancer research tasks run on World Community Grid, as well as the first sets of ovarian cancer data. This update gives a detailed look at the tools and processes they are using for this analysis, plus a list of their recent publications and events in their lab.
The Mapping Cancer Markers project aims to identify chemical markers associated with various types of cancer. This will help researchers detect cancer earlier and design more personalized cancer care. Below, the research team describes how they are analyzing the lung cancer data from research tasks that were run on World Community Grid, as well as the first sets of ovarian cancer data. They also update us on happenings in their lab, and provide information on their recent publications.
Lab news
Dr. Anne-Christin Hauschild has recently joined our lab as a postdoctoral research fellow, and will be contributing to the Mapping Cancer Markers project by applying data mining and machine learning algorithms to further prioritize signatures and characterize involved genes.
Our work was recognized for the third time in the row by Thomson Reuters, who included us in the highly cited researcher list, out of 127 in computer science and 3,266 world-wide in 21 fields of science.
Transition to the ovarian dataset
Our previous update described the planned transition to an ovarian cancer dataset from the lung cancer analysis. Due to the timing of the ovarian dataset tests and their launch on World Community Grid, we had several extra, unscheduled days of lung cancer analysis. We used these extra days to explore larger lung cancer signatures (30-100 markers). Previous Mapping Cancer Markers lung cancer work units explored smaller signatures (5-25 markers). All lung cancer results have since been collected, along with the first few months of ovarian cancer results. We are hard at work analyzing the completed lung and preliminary ovarian results.
How we are processing results
As part of this work, we have overhauled how we handle and process the results we receive from World Community Grid. Specifically, we have changed our Extract-Transform-Load (ETL) system which takes the raw, packaged research tasks received from World Community Grid, and unpacks, collates, reorganizes, and recodes results into an efficient and easy-to-load format for subsequent analyses. Our previous ETL system was built into our IBM InfoSphere Streams analysis pipeline. Separating the ETL stage from the analysis benefits the project in several ways. It allows us to more efficiently store data, it simplifies our main Streams-based analysis pipeline, and most importantly, it allows direct analysis of MCM results with other tools and platforms (such as data mining and data analysis tools like R and scikit-learn).
Minimizing potential bias in the ovarian cancer dataset
The Mapping Cancer Markers ovarian cancer dataset combines data from multiple, independent cancer studies. These studies did not follow identical protocols in selecting patients, tissue sample collection or preparation, or recording of clinical covariates. Combining data from multiple sources together requires careful normalization (the process of reorganizing data). The search for successful signatures in such a dataset is made easier if the dataset minimizes bias. We will continue to study the issue of data normalization in the ovarian cancer dataset, and may update the dataset in the future if we discover improvements, or if analysis of results reveals biases.
Data integration portals
Our team has developed two data integration portals to help us interpret and validate the results we receive from Mapping Cancer Markers. The functions of these two portals, called mirDIP and pathDIP, are described in detail below.
Using mirDIP to interpret Mapping Cancer Markers results
One of the projects that our group has been working on in the past several months is the MicroRNA Data Integration Portal (mirDIP). This web resource allows users to query MicroRNAs (miRNAs) and to investigate their interactions with messenger RNA (mRNA) targets. MicroRNAs are short and non-coding RNA molecules observed across plants, animals and viruses(1). For the most part, these short molecules bind to mRNA to control the quantity of protein production. For example, in the case where someone might be injured and is bleeding, if the body determines that there is an immediate need for more blood, miRNAs may bind to their targets and turn up the production of the protein hemoglobin. These molecules are important in the study of cancer because, in part, they control when things should be turned on or off. MiRNAs are known to target more than one gene, so understanding their relationships with genes is an important step in understanding how genes and proteins function.
One of the ways that we plan to use mirDIP in tandem with our Mapping Cancer Markers project is to look more closely at common genes identified by the two methods. Below, we use an example of how this is done. If we take one of many publications to start(2), we can identify miRNAs that are known to be related to ovarian cancer. As seen in a figure from the publication, we can identify some of the key players involved. Networks such as this provide valuable information, yet by no means completely characterize the environment.
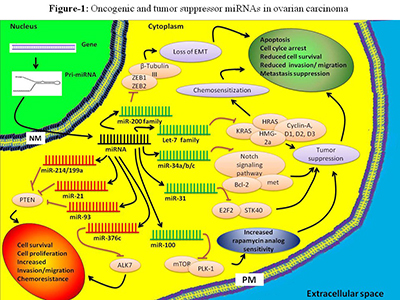
Figure 1. Oncogenic and tumor suppressor miRNAs in ovarian carcinoma. Based on their function, miRNAs can be used for diagnostics and therapeutics. Certain miRNAs such as miR-200 family, let-7 family, miR-21, miR-214, and miR-100 have strong diagnostic/prognostic potential in ovarian cancer. Figure and caption from paper by Zaman et al. (2), licensed under CC BY 2.0.
We can use mirDIP to identify other major and minor genes that may be involved in these processes. If we submit a query using the 8 example miRNAs, and limit results to those referenced in at least 5 databases, we can quickly narrow down a list of genes of interest. Here, we show an analysis using the software package we developed for visualizing and analyzing protein-protein interaction networks (NAViGaTOR 3.0) of 8 miRNAs and associated genes, where at least 2 miRNAs are targeting a gene.
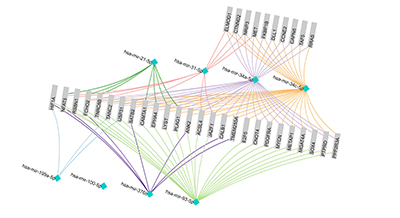
Figure 2. NAViGaTOR 3.0 network of ovarian cancer-associated miRNAs and genes. Turquois nodes indicate miRNAs and grey boxes indicate associated genes, predicted by our mirDIP portal. Two of the miRNAs hsa-mir-34a-5p and has-mir-34c-5p are related to each other and thus have a high amount of overlap across genes they regulate. Hsa-mir-100-5p has no interacting genes; however, this network only shows interactions validated by over 5 independent databases. Hsa-mir-100-5p interactions may only have been significantly identified using fewer sources.
In turn, those genes may indicate critical pathways (some of which are identified in Figure 1) or novel pathways, which may be compromised. If we compare the results of our mirDIP analysis to our highest-scoring Mapping Cancer Markers signatures, we can further identify particular genes of interest. Understanding which players (pathways, genes, proteins, miRNAs, etc) are involved and predicting the possible mechanism will lead to focusing further studies, and may lead to identifying targeted treatment for specific patient subgroups—the goal of precision medicine.
Systematic and comprehensive pathway analysis using pathDIP
This brings us to another resource we have created for comprehensive characterization of cancer profiles: pathDIP. Importantly, this public resource integrates 20 databases and enables computational prediction of pathway association, necessary step to fully understand signal cascades in healthy and disease conditions. Cross-validation determined 71% accuracy of our predictions, and predictions provide novel annotations for 5,732 proteins previously lacking pathway characterization.
Taking the results from Figure 2 (the 36 genes identified by mirDIP), the pathDIP portal identifies two significantly enriched pathways (p < 0.05): 1) MicroRNAs in cancer, and 2) Central carbon metabolism in cancer (KEGG database). The first pathway directly confirms our steps to this point while the latter pathway indicates another avenue for exploration. Indeed, the central carbon metabolism pathway includes the conversion of glucose to lactic acid, a process known as the Warburg effect, which is common in ovarian and other cancers. This process has been shown to be controlled by nitric oxide (3) as well as other miRNAs (4).
Studying broccoli’s anti-cancer properties
However, going back to microRNA gene regulation – the mirDIP portal now enables us to study whether microRNAs from animals, plants and viruses could regulate human genes. This cross-species regulation mechanism opens enormous potential for understanding increase in disease risk and prevention. As a first study in this direction, we have recently completed and published a paper showing that broccoli microRNAs do regulate human genes, upregulated in lung cancer, thus providing a potential explanation of why broccoli consumption has been linked to anti-cancer properties by many epidemiological studies.
- Pastrello, C., Tsay, M., McQuaid, R., Abovsky, M., Pasini, E., Shirdel, E., Angeli, M., Tokar, T., Jamnik, J., Kotlyar, M., Jurisicova, A., Kotsopoulos, J., El-Sohemy, A., Jurisica, I. Circulating plant miRNAs can regulate human gene expression in vitro. Nat Sci Reports 6: 32773, 2016.
Several related publications
While most of these publications are related to either cancer studies or tools and resources we created, we also continue to collaborate with other researchers and translate verified workflows from cancer informatics to help solve other diseases.
Here are some of our recent publications:
- Chehade, R., R. Pettapiece-Phillips, Salmena, L., Kotlyar, M., Jurisica, I., Narod, S. A., Akbari, M. R., Kotsopoulos, J. Reduced BRCA1 transcript levels in freshly isolated blood leukocytes from BRCA1 mutation carriers is mutation specific, Breast Cancer Res, 18(1): 87, 2016.
- Cierna, Z., Mego, M., Jurisica, I., Machalekova, K., Chovanec, M., Miskovska, V., Svetlovska, D., Hainova, K., Kajo, K., Mardiak, J., Babal, P. Fibrillin-1 (FBN-1) a new marker of germ cell neoplasia in situ, BMC Cancer, 16: 597, 2016.
- Nakamura, A., Rampersaud, R., Sharma, A., Lewis, S.J., Wu, B., Datta,P., Sundararajan, K., Endisha, H., Rossomacha, E., Rockel, J.S., Jurisica, I., Kapoor, M., Identification of microRNA-181a-5p and microRNA-4454 as mediators of facet cartilage degeneration, JCI Insight, 1(12):e86820, 2016.
- Becker-Santos, D.D., Thu, K.L, English, J.C., Pikor, L.A., Chari, R., Lonergan, K.M., Martinez, V.D., Zhang, M., Vucic, E.A., Luk, M.T.Y., Carraro, A., Korbelik, J., Piga, D., Lhomme, N.M., Tsay, M.J., Yee, J., MacAulay, C.E., Lockwood, W.W., Robinson, W.P., Jurisica, I., Lam, W.L., Developmental transcription factor NFIB is a putative target of oncofetal miRNAs and is associated with tumour aggressiveness in lung adenocarcinoma, J Pathology, In press.
- Konvalinka, A., Batruch, I., Tokar, T., Dimitromanolakis, A., Reid, R., Song, X., Pei, Y., Drabovich, A.P.,PhD; Diamandis, E. P., Jurisica, I., Scholey, J.W. Quantification of Angiotensin II-Regulated Proteins in Urine of Patients with Polycystic and Other Chronic Kidney Diseases by Selected Reaction Monitoring, Clinical Proteomics, 13: 16, 2016.
- Stojanova, A., Tu, W.B., Ponzielli, R., Kotlyar, M., Chan, P.K., Boutros, P.C., Khosravi, F., Jurisica, I., Raught, B., Penn, L.Z. MYC interaction with the tumor suppressive SWI/SNF complex member INI1 regulates transcription and cellular transformation, Cell Cycle, 15(13): 1693-705, 2016.
- Li, Y-H, Tavallaee, G., Tokar, T., Nakamura, A., Sundararajan, K., Weston, A., Sharma, A., Mahomed, N. N., Gandhi, R., Jurisica, I., Kapoor, M. Identification of synovial fluid microRNA signature in knee osteoarthritis: Differentiating early- and late-stage knee Osteoarthritis. Osteoarthritis and Cartilage, 24(9): 1577-86, 2016.
- Cinegaglia, N.C., Andrade, S.C.S., Tokar, T., Pinheiro, M., Severino, F. E., Oliveira, R. A., Hasimoto, E. N., Cataneo, D. C., Cataneo, A.J.M., Defaveri, J., Souza, C.P., Marques, M.M.C, Carvalho, R. F., Coutinho, L.L., Gross, J.L., Rogatto., S.R., Lam, W.L., Jurisica, I., Reis, P.P. Integrative transcriptome analysis identifies deregulated microRNA-transcription factor networks in lung, adenocarcinoma, Oncotarget, 7(20): 28920-34, 2016.
- Vargas, A., Angeli, M., Pastrello, C., McQuaid, R., Li, H., Jurisicova, A., Jurisica, I., Robust quantitative scratch assay, Bioinformatics, 32(9):1439-40, 2016.
Other news
We have completed the 6th annual Team Ian Ride, raising funds to support training of young researchers. These funds support the Best Student Paper Award at the annual ISMB conference, as well as summer interns in cancer informatics.
The event also supports a new direction of our research into physical activity and cancer prevention, and many Team Ian participants have already enrolled in our experimental studies. You can find images at http://www.cs.utoronto.ca/~juris/TIR2016. Please contact us if you want to get involved in 2017.
Thank you,
Mapping Cancer Markers team
References
1. He,L. and Hannon,G.J. (2004) MicroRNAs: small RNAs with a big role in gene regulation. Nat. Rev. Genet., 5, 522–531.
2. Zaman,M.S., Maher,D.M., Khan,S., Jaggi,M., Chauhan,S.C., Siegel,R., Naishadham,D., Jemal,A., Wright,J., Shah,M., et al. (2012) Current status and implications of microRNAs in ovarian cancer diagnosis and therapy. J. Ovarian Res., 5, 44.
3. Caneba CA, Yang L, Baddour J, Curtis R, Win J, Hartig S, Marini J, Nagrath D. (2014) Nitric oxide is a positive regulator of the Warburg effect in ovarian cancer cells. Cell Death Dis. 5:e1302.
4. Yue Teng, Yan Zhang, Kai Qu, Xinyuan Yang, Jing Fu, Wei Chen, and Xu Li. (2015) MicroRNA-29B (mir-29b) regulates the Warburg effect in ovarian cancer by targeting AKT2 and AKT3. Oncotarget. 6(38): 40799-S40814.
Related Articles
- Project roadmap and first phase results from the Mapping Cancer Markers team
- Early-stage results from the Mapping Cancer Markers team
- Using one cancer to help defeat many: Mapping Cancer Markers makes progress
- One step closer to identifying lung cancer biomarkers
- Working to detect lung and ovarian cancers before they start
- Mapping Cancer Markers Now Examining Ovarian Cancer
- Mapping Cancer Markers Team Analyzes Lung Cancer Data
- New Facility and Expanded Plans for the Mapping Cancer Markers Research Team
- Sarcoma Dataset Coming Soon to Mapping Cancer Markers Project